Researchers Develop Forensic Techniques to Identify ‘Deepfakes’
“DEEPFAKES” — DOCTORED VIDEOS that appear to show a person doing or saying something when in fact they did not — are finding their way into public view. So alarming is the potential for the widespread of potentially dangerous misinformation that scientists have been working to identify such videos more quickly.
Deepfakes were discussed in congressional testimony in June, around the same time a doctored video of Facebook CEO Mark Zuckerberg began circulating in which he appeared to talk about the potential use of data. Many people are concerned about the forthcoming 2020 elections in the United States and how deepfakes could misrepresent a candidate’s statements, for example, as well as the potential of deepfakes to cause conflict on a global stage.
Computer scientists from the USC Information Sciences Institute (ISI), including Ekraam Sabir, Jiaxin Cheng, Ayush Jaiswal, Wael Abd-Almageed, Iacopo Masi and Prem Natarajan, have been working on ways to detect manipulated content. Their paper was presented recently at the IEEE Conference on Computer Vision and Pattern Recognition in Long Beach, California.
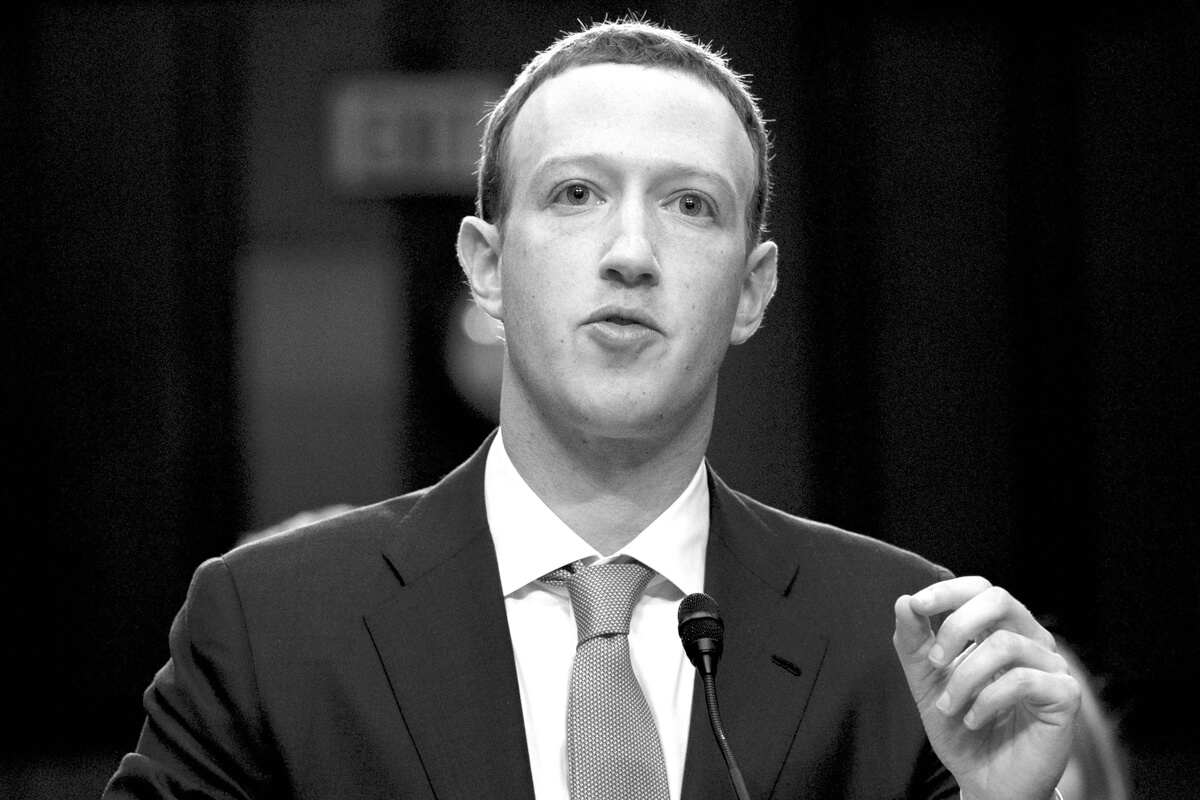
In “Recurrent Convolutional Strategies for Face Manipulation Detection in Videos,” they described a method to identify deepfakes that performs with 96 percent accuracy when evaluated on the only large-scale deepfake data set called FaceForensics++. Their method works on various types of content manipulations known as deepfakes, face-swaps and face2face, even after being highly compressed to hide the manipulation artifacts. At the point of publishing, the authors said their detection method was ahead of the content manipulators, who quickly modify as new detection methods arise.
While previous methods of detecting deepfakes typically use frame-by-frame analysis of a video, the researchers contend that these techniques are computationally heavy, take more time and leave greater room for error. The newer tool developed by ISI, which was tested on more than 1,000 videos, is less computationally intensive and has the potential to scale and automatically detect fakes that are uploaded in the millions of profiles on Facebook or other social media platforms in near-real-time.
Led by principal investigator Wael Abd-Almageed, a computer vision, facial recognition and biometrics expert at USC Viterbi, it looks at a piece of video content as a whole. The researchers used AI to identify inconsistencies in the video frames through time, not just on a frame-by-frame basis. Using a deep learning algorithm known as a convolutional neural network, the researchers identified features and patterns in a person’s face, with specific attention to how the eyes close or the mouth moves. Once they had a model for human faces and the movements surrounding their facial movements, they could develop a tool that compares a newly input video with the parameters of the previous models to determine if a piece of content was outside the norm and thus not authentic.
“If you think deepfakes, as they are now, are a problem, think again. Deepfakes, as they are now, are just the tip of the iceberg, and manipulated video using artificial intelligence methods will become a major source of misinformation,” Abd-Almageed said.